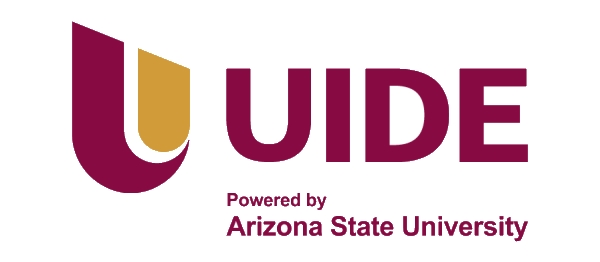
Please use this identifier to cite or link to this item:
https://repositorio.uide.edu.ec/handle/37000/6126
Full metadata record
DC Field | Value | Language |
---|---|---|
dc.contributor.author | Moya, Oscar | - |
dc.contributor.author | Aguilar, Edison | - |
dc.contributor.author | Palacios, Santiago | - |
dc.contributor.author | Tipán, Gabriela | - |
dc.contributor.author | Vizcaíno, Paulina (tutor) | - |
dc.date.accessioned | 2023-07-26T21:16:50Z | - |
dc.date.available | 2023-07-26T21:16:50Z | - |
dc.date.issued | 2023 | - |
dc.identifier.citation | Moya, Oscar; Aguilar, Edison; Palacios, Santiago; Tipán, Gabriela. (2023). Diseño de un modelo de prevención de fraudes en transacciones digitales con técnicas de machine learning. Facultad de Ciencias Técnicas. UIDE. Quito. 65 p. | es |
dc.identifier.other | UIDE-Q-TMSIG-2023-10 | - |
dc.identifier.uri | https://repositorio.uide.edu.ec/handle/37000/6126 | - |
dc.description.abstract | Uno de los principales riesgos a los que está sometido el e-commerce dentro de la industria financiera, telecomunicaciones, entre otras, son los ataques de fraudes electrónicos, producto de transacciones digitales. Por tal motivo es fundamental implementar mecanismos que ayuden a prevenir de manera efectiva características anómalas que conlleven al cometimiento de fraudes electrónicos y pérdidas dentro de la organización. El presente proyecto plantea un modelo para prevención de fraudes en transacciones digitales con técnicas de machine learning, basado en datos proporcionados por la organización, la cual pertenece a la industria de telecomunicaciones, de quien guardamos confidencialidad por la sensibilidad de la información trabajada en el modelo. El modelo planteado utiliza datos de transacciones digitales del año 2021 y primer semestre del 2022 debido a que, en los años mencionados, existió un incremento considerable de transacciones fraudulentas, las cuales conllevaron a pérdidas económicas significativas e incremento del número de contracargos reportados por las entidades adquirentes. Una de las finalidades de este proyecto, es que el área de control de fraudes cuente con una herramienta que facilite la detección de transacciones fraudulentas de manera oportuna y a la vez la toma de decisiones en las áreas de negocio para mitigar el riesgo de pérdidas dentro de la organización y promover el aseguramiento de ingresos. One of the main risks to which e-commerce is subjected within the financial industry, telecommunications, among others, are electronic fraud attacks, a product of digital transactions. For this reason, it’s essential to implement mechanisms that help to effectively prevent anomalous characteristics that contribute to the commission of electronic fraud and losses within the organization. The present project plans a model for the prevention of fraud in digital transactions with machine learning techniques, based on data provided by the organization, which belongs to the telecommunications industry, which we keep confidential due to the sensitivity of the information worked in the model. The proposed model uses data from digital transactions from the year 2021 and the first half of 2022 due to the fact that in the years mentioned, there was a considerable increase in fraudulent transactions, which led to significant economic losses and an increase in the number of charges reported by the acquiring entities. One of the purposes of this project is that the fraud control area uses a tool that facilitates the detection of fraudulent transactions in a timely manner and at the same time makes decisions in the business areas to mitigate the risk of losses within the organization and promoting income security. | es |
dc.language.iso | es | es |
dc.publisher | QUITO/UIDE/2023 | es |
dc.rights | openAccess | es |
dc.subject | MODELO DE PREVENCIÓN | es |
dc.subject | FRAUDES | es |
dc.subject | TRANSACCIONES DIGITALES | es |
dc.subject | TÉCNICAS DE MACHINE LEARNING | es |
dc.title | Diseño de un modelo de prevención de fraudes en transacciones digitales con técnicas de machine learning | es |
dc.type | Thesis | es |
Appears in Collections: | Tesis - Maestría - Sistemas de Información |
Files in This Item:
File | Description | Size | Format | |
---|---|---|---|---|
UIDE-Q-TMSIG-2023-10.pdf | CONFIDENCIAL | 153.72 kB | Adobe PDF | View/Open |
Items in DSpace are protected by copyright, with all rights reserved, unless otherwise indicated.